Phantom of the ADAS
Securing Advanced Driver Assistance Systems from Split-Second Phantom Attacks
Ben Nassi* Yisroel Mirsky* Dudi Nassi*
Raz Ben Netanel* Green** Yuval Elovici*
*Ben-Gurion University of the Negev **Independent Tesla Researcher
TLDR
We were able to trigger Tesla's autopilot to stop in the middle of the road in
response to a 125 ms stop sign that was added to an advertisement presented
on a digital billboard.
​
Abstract
In this paper, we investigate "split-second phantom attacks," a scientific gap that causes two commercial advanced driver-assistance systems (ADASs), Telsa Model X (HW 2.5 and HW 3) and Mobileye 630, to treat a depthless object that appears for a few milliseconds as a real obstacle/object. We discuss the challenge that split-second phantom attacks create for ADASs. We demonstrate how attackers can apply split-second phantom attacks remotely by embedding phantom road signs into an advertisement presented on a digital billboard which causes Tesla’s autopilot to suddenly stop the car in the middle of a road and Mobileye 630 to issue false notifications. We also demonstrate how attackers can use a projector in order to cause Tesla’s autopilot to apply the brakes in response to a phantom of a pedestrian that was projected on the road and Mobileye 630 to issue false notifications in response to a projected road sign. To counter this threat, we propose a countermeasure which can determine whether a detected object is a phantom or real using just the camera sensor. The countermeasure (GhostBusters) uses a "committee of experts" approach and combines the results obtained from four lightweight deep convolutional neural networks that assess the authenticity of an object based on the object’s light, context, surface, and depth. We demonstrate our countermeasure’s effectiveness (it obtains a TPR of 0.994 with an FPR of zero) and test its robustness to adversarial machine learning attacks.
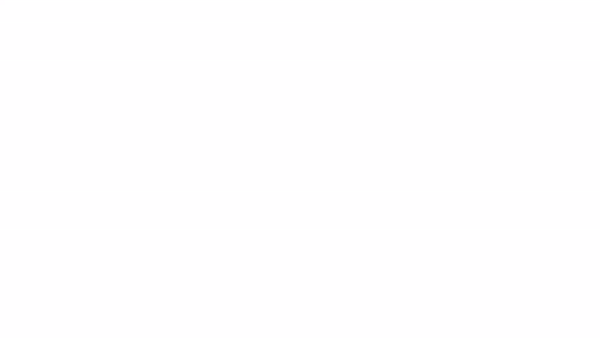
Associated Publications
-
Protecting Autonomous Cars from Phantom Attacks (Communications of the ACM)
-
Phantom of the ADAS - Securing Advanced Driver-Assistance Systems from Split-Second Phantom Attacks (CCS'20)
-
Spoofing Mobileye 630’s Video Camera Using a Projector (AutoSec'21)
-
Phantom of the ADAS: Phantom Attacks on Driver-Assistance Systems (IACR)
The Perceptual Challenge
Would you consider the projection of the person and road sign real?
Telsa considers the projected character as a real person.
Mobileye 630 PRO considers the projected road sign as a real road sign.

Phantoms
We define a phantom as a depthless visual object used to deceive ADASs and cause these systems to perceive the object and consider it real. A phantom object can be created by a projector or be presented via a digital screen (e.g., billboard). The depthless object presented/projected is made from a picture of a 3D object (e.g., pedestrian, car, truck, motorcycle, traffic sign). The phantom is intended to trigger an undesired reaction from an ADAS.
For example, the picture below presents a projected phantom of a car that was detected by the
Tesla (HW 2.5) which considered it a real car.
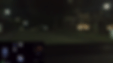
Split-Second Phantom Attacks
A split-second phantom attack is a phantom that appears for a few milliseconds and is treated as a real object/obstacle by an ADAS.
​
​
​
​
​
​
​
​
What is the minimal duration that a phantom needs to appear in order to be detected by ADAS?
​
​
​
​
​
​
​
​
Split Second Phantom Attacks can be applied via advertisement and cause ADAS to trigger a reaction. Attackers can use a dedicated algorithm to hide a phantom in an arbitrary advertisement:
​
Split Second Phantom Attack applied Against Tesla Model X (via digital billboard)
Split Second Phantom Attack applied Against Mobileye 630 PRO (via digital billboard)
​
​
​
​
​
​
​
​
​
Split Second Phantom Attack applied Against Mobileye 630 PRO (via a projector)



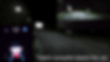
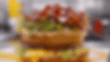

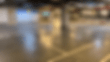
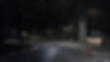
Talks
Media
FAQ
​​
Are phantoms bugs?
No. Phantoms are definitely not bugs.
They are not the result of poor code implementation in terms of security.
They are not classic exploitation (e.g., buffer overflow, SQL injections) that can be
easily patched by adding an "if" statement.
They reflect a fundamental flaw of models that detect objects that were not trained to distinguish
between real and fake objects.
​
Why are phantom attacks so dangerous?
Previous attacks:
1. Necessitate that the attackers approach the attack scene in order to manipulate an object
using a physical artifact (e.g., stickers, graffiti) or to set up the required equipment,
acts that can expose attackers’ identities.
2. Require skilled attackers (experts in radio spoofing or adversarial machine learning techniques).
3. Required full knowledge of the attacked model.
4. Leave forensic evidence at the attack scene.
5. Require complicated/extensive preparation (e.g., a long preprocessing phase to
find an evading instance that would be misclassified by a model).
​
Phantom attacks:
1. Can be applied remotely (using a drone equipped with a portable projector or by hacking digital billboards that face the Internet and are located close to roads), thereby
eliminating the need to physically approach the attack scene, changing the exposure vs.
application balance.
2. Do not require any special expertise.
3. Do not rely on a white-box approach.
4. Do not leave any evidence at the attack scene.
5. Do not require any complex preparation.
6. Can be applied with cheap equipment (a few hundred dollars).
​​
Why does Tesla consider phantoms real obstacles?
We believe that this is probably the result of a "better safe than sorry" policy that considers a visual projection a real object even though the object is not detected by other sensors (e.g., radar and ultrasonic sensors).
​​
Can phantoms be classified solely based on a camera?
Yes.
By examining a detected object's context, reflected light, and surface,
we were able to train a model that accurately detects phantoms (0.99 AUC).
​
Will the deployment of vehicular communication systems eliminate phantom attacks?
No.
The deployment of vehicular communication systems might limit the opportunities
attackers have to apply phantom attacks, but won’t eliminate them.
​​
Did you disclose your findings to Mobileye and Tesla?
Yes.
We kept Tesla and Mobileye updated via a series of emails sent from early May to October 19.